Research: automatic machine learning turbine optimisation is coming
Wind turbines operate in very different conditions, even on the same site, and optimising each turbine to actual conditions can therefore have a considerable impact on the energy yield. Continuous turbine optimisation seems possible through the use of machine learning (ML) solutions that will enable safe, dependable, automatic, continuous, and individualised turbine optimisation. DEIF has achieved encouraging preliminary results with yaw positioning in a test project.
All over the world, wind turbines operate in very different conditions. Climate factors and wind farm phenomena such as wake effects mean that otherwise identical turbines perform very differently, even on the same site. Carefully optimising each individual turbine to actual onsite conditions in order to maximise the usage of the potential wind energy yield can therefore have a considerable impact on the AEP and lifetime of the turbines.
However, modern turbine controllers have hundreds of parameters that can be adjusted, and turbines are therefore rarely optimised and fine-tuned continuously and individually in order to adjust to changing conditions: it is too time-consuming and requires expert knowledge of both the turbine controller and wind farm conditions.
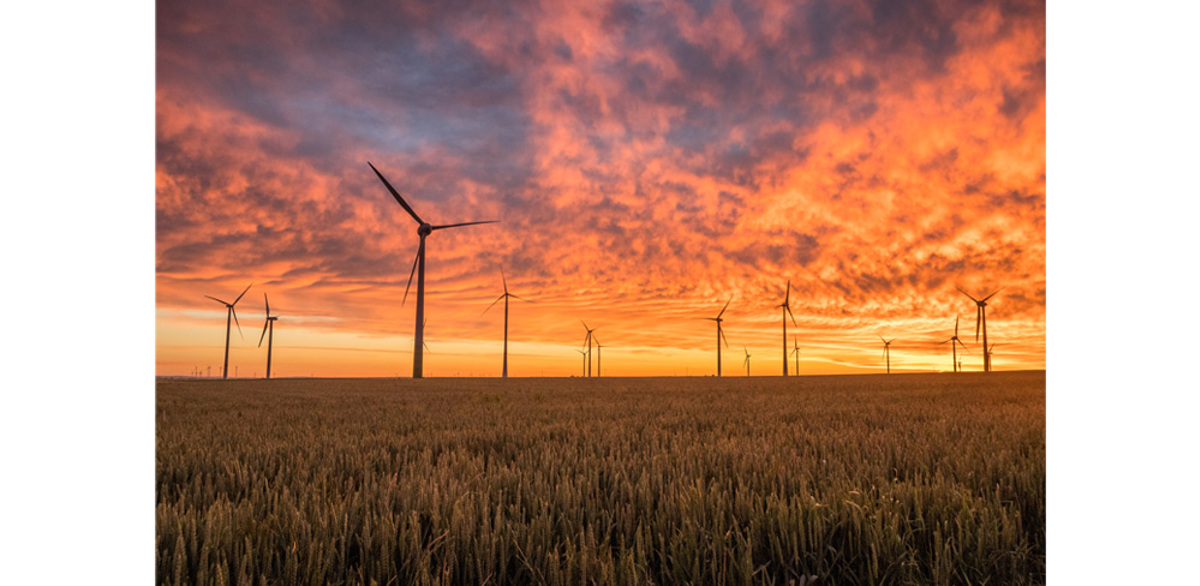
Wind turbines perform very differently depending on actual operating conditions, even identical turbines on the same wind farm. Machine learning and cloud computing could soon allow continuous, automatic, and individualised turbine optimisation based on actual operating conditions.
Continuous turbine optimisation, however, seems to be possible through the use of machine learning (ML). DEIF is currently involved in research aiming to develop robust ML solutions that will enable safe, dependable, automatic, continuous, and individualised turbine optimisation.
How does it work?
The idea is to gather data about individual turbine operation and environmental conditions and using that data to find the optimal parameter settings for each individual turbine. The settings are then transferred back to the turbine, adjusting it for maximised power production under the given environmental conditions. In brief, this is how it works:
- Raw data from turbines is gathered in 10-minute aggregates. In addition, high-speed data is collected where possible, allowing the system to optimise parameters that require higher sampling rates in order to observe the turbine state.
- Based on the data collected, algorithms based on reinforcement learning (a category of machine learning) are trained to maximise the turbine’s power output, while minimising the loads at the same time. Calculations are made in a powerful cloud computing environment set up to simulate conditions and turbine operation. To ensure the operational and structural safety of the wind turbine, the agent must consider load calculations when optimising some turbine parameters.
- When the agent is sufficiently trained, it becomes stable. At this point, the simulation environment starts taking in observations directly from a live turbine, and it carries out parameter changes directly on that turbine. As a result, the turbine is now continuously adjusted based on live data, maximising its performance and yielding the best possible power production.
Encouraging preliminary results
Continuous turbine adjustment through ML sounds good in theory, but will it work in practice? To test the concept, DEIF recently developed a reinforcement learning agent designed to minimise turbine power losses caused by non-optimal yaw positioning relative to the wind direction. Early results indicated that the agent could learn to optimise a yaw offset parameter in less than 5,000 observations.
While the model used was simpler than the complex models required to carry out optimisation of all turbine parameters, it proved that the concept itself is viable. More research into complex models and parameters will be required. DEIF has received a grant from the Austrian Research Institution (FFG) to continue our research on this topic over the next 3 to 5 years. Hopefully, it will contribute to more robust and production-ready agents that can be deployed to operate turbines closer to their theoretical limits through safe, dependable, automatic, continuous, and individualised turbine optimisation.
Get more information about DEIF retrofit solutions for wind turbines